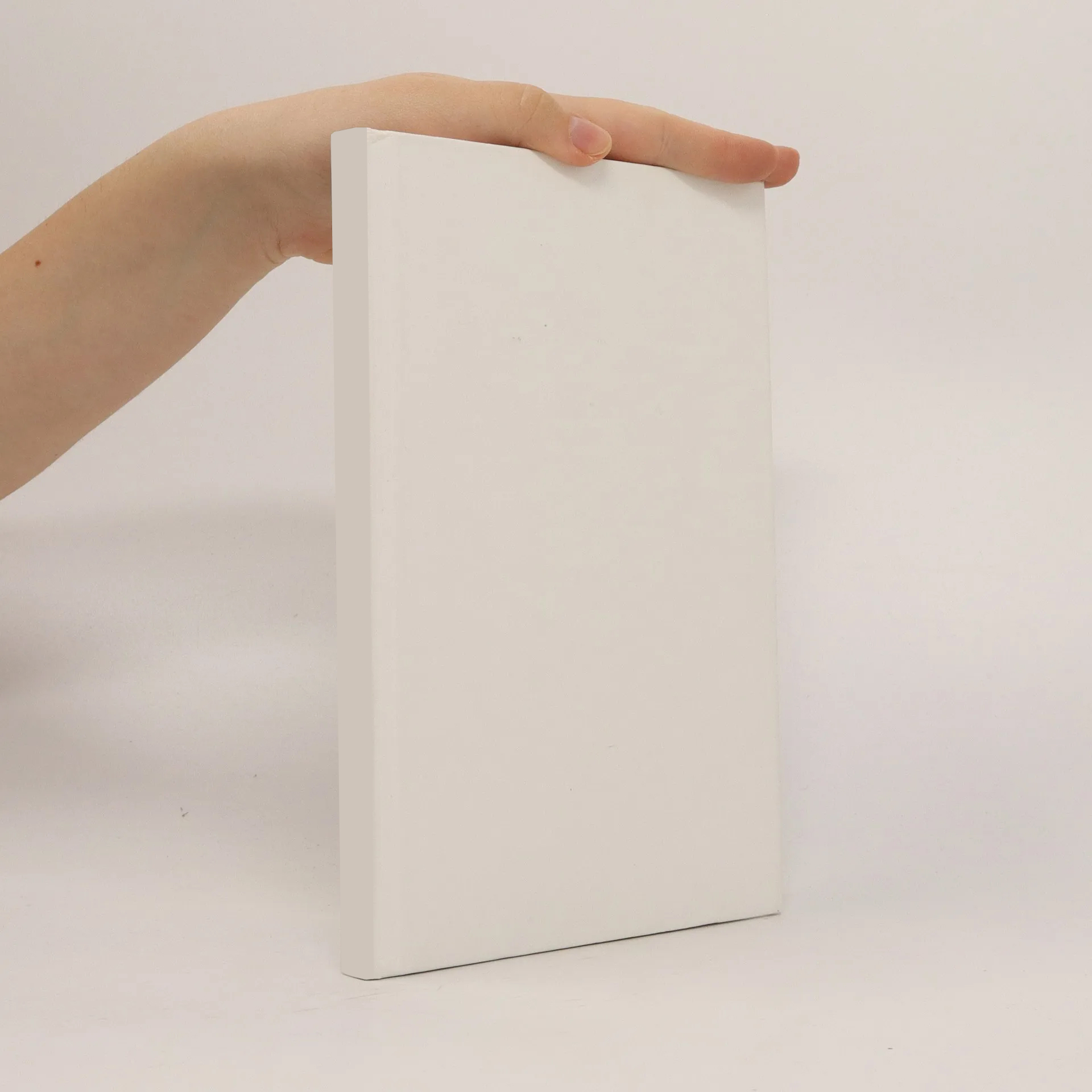
Více o knize
The research of methods equipping a technical system with the ability to learn mental concepts of objects, properties, or actions is an important step towards the understanding of intelligence. A special challenge arises from the variety of different characteristics whose association forms our understanding of a concept. For example the concept of a table might comprise a decisive collection of planarity, height, size, the action of placing something on the surface, and the speech label used to refer to the table. In the last decade the growing awareness that concepts must be linked to the real world has led to several approaches capable of learning concepts from interaction. However, most of these systems require supervision during the learning process; others lack the scalability required to span the variety of possible associations forming a concept. An important research question that has been vastly neglected concerns the visual perception: How can a system segregate objects from its surrounding, if it lacks any knowledge about their appearance? In recent approaches this question has been avoided by constraining the learning scenario to more or less static platforms observing objects on a uniformly colored table. This does not only limit the concepts to be learned but also prevents natural interaction. The contribution of this work covers these three different aspects: It presents an unsupervised mechanism for the leaming of multimodal concepts, a generic framework for visual perception linking these concepts to the real world, and a system embedding the above on a humanoid robot acting autonomously in dynamic scenes.
Nákup knihy
Multimodal learning of grounded concepts in embodied systems, Jens Martin Schmu dderich
- Jazyk
- Rok vydání
- 2010
Doručení
Platební metody
Nikdo zatím neohodnotil.