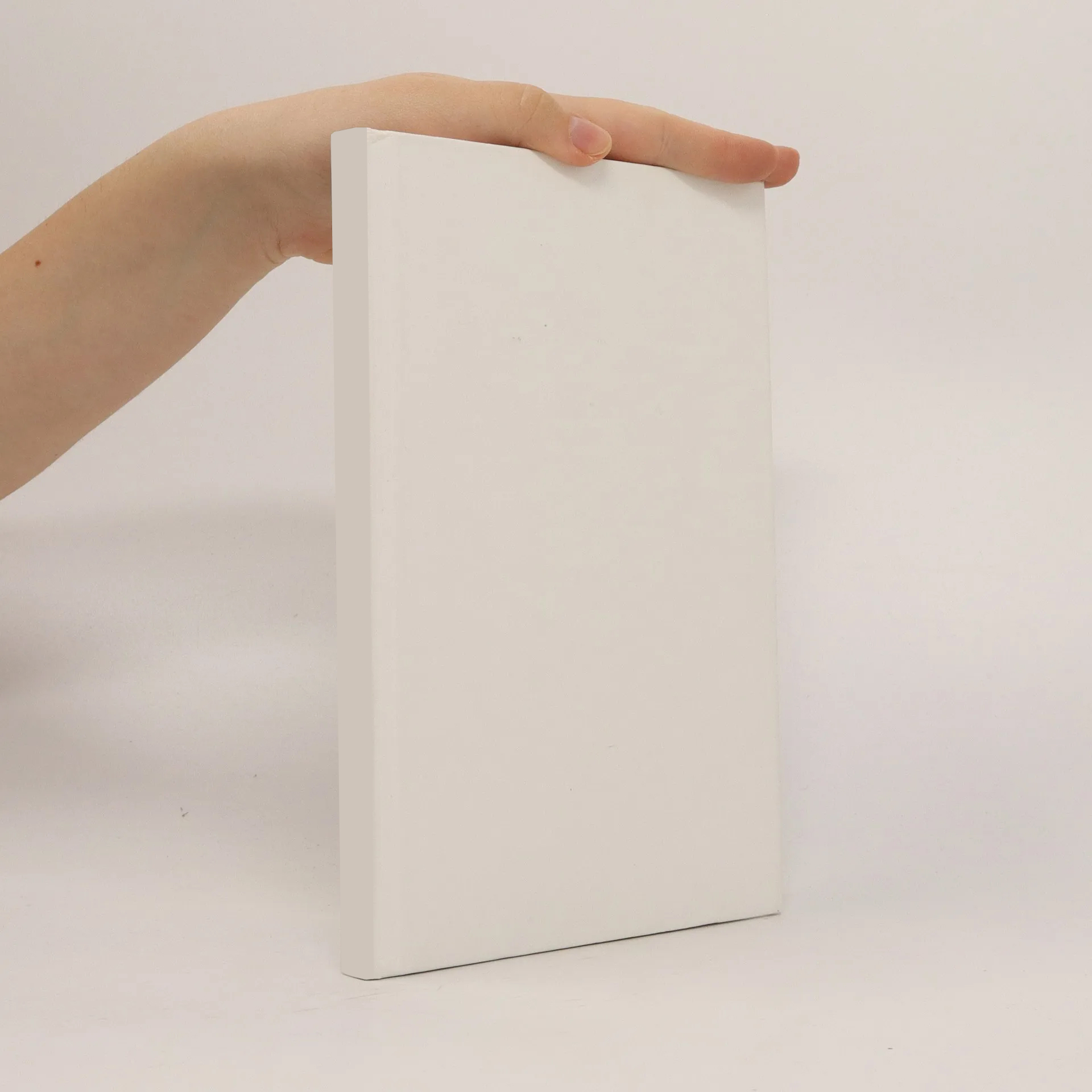
Data- and model-based identification of biochemical processes
Autoři
Více o knize
In the last decade a paradigm shift has taken place in biochemical research: while traditionally biochemical processes have often been studied on a qualitative level, more and more research now focuses on quantitative time-resolved aspects of biochemical processes. However, on a quantitative dynamic level the complexity of these processes increases significantly and the need of mathematical models arises. Once a model is fitted to experimental data it can be used to simulate and study the dynamic behavior of a given process. Furthermore, a fitted model allows it to test new experiments and hypothesis in silico before time and cost intensive real experiments need to be conducted. The interplay between biochemical experimentation and mathematical modeling - known as systems biology - is an integral part of this thesis. Identifying a predictive model starts with the formulation of an initial model, which combines a priori knowledge with new to be tested hypotheses. The initial model is refined in an iterative process of performing quantitative experiments, estimating unknown model parameters, model validation and hypothesis testing. When constructing a model, it is tempting to incorporate all known interactions between biochemical species, which results in models with a large number of unknown parameters, which subsequently have to be estimated from experimental data. However, parameter estimation can only provide valid results, if the complexity of the model and the amount and quality of data are in balance with one another. If this is the case the model is said to be identifiable for the given data. In Chapter 2 of this thesis we describe a new automatic approach to test the identifiability of model parameters. We compare our new method - the eigenvalue method - to three well established methods for identifiability testing. For three published models of signaling cascades our eigenvalue methods outperforms the other methods in terms of efficiency and effectiveness. Furthermore, we find that even when assuming abundant and noise-free measurement data, the three models are not identifiable. If a model turns out to be unidentifiable, two steps can be taken. Either additional experiments need to be conducted to increase the information content of the data, or the model has to be simplified. In Chapter 3 we follow the latter path and describe an iterative approach that combines multi-start parameter estimation, identifiability testing, sampling-based variance analysis and goodness-of-fit testing into a work flow for model simplification. We demonstrate the effectiveness of this work flow by simplifying a published model of a signaling cascade under the assumption of realistic measurements until a good fitting model with identifiable and barely varying parameters results. Finally, in Chapter 3 we demonstrate the power of a data-driven model-based approach for process identification by discriminating between different hypotheses on the function of SHP2 in the early phase of JAK-STAT signaling. Furthermore, we identify key processes that are essential for the dynamics of early pathway activation. In addition to the techniques presented in Chapters 1 and 2 we apply a brute-force method for optimal experimental design to propose new informative experiments. Using an initial and the optimal designed data, we iteratively refine our model until an identifiable and predictive model of early JAK-STAT signaling results that adequately describes the data.