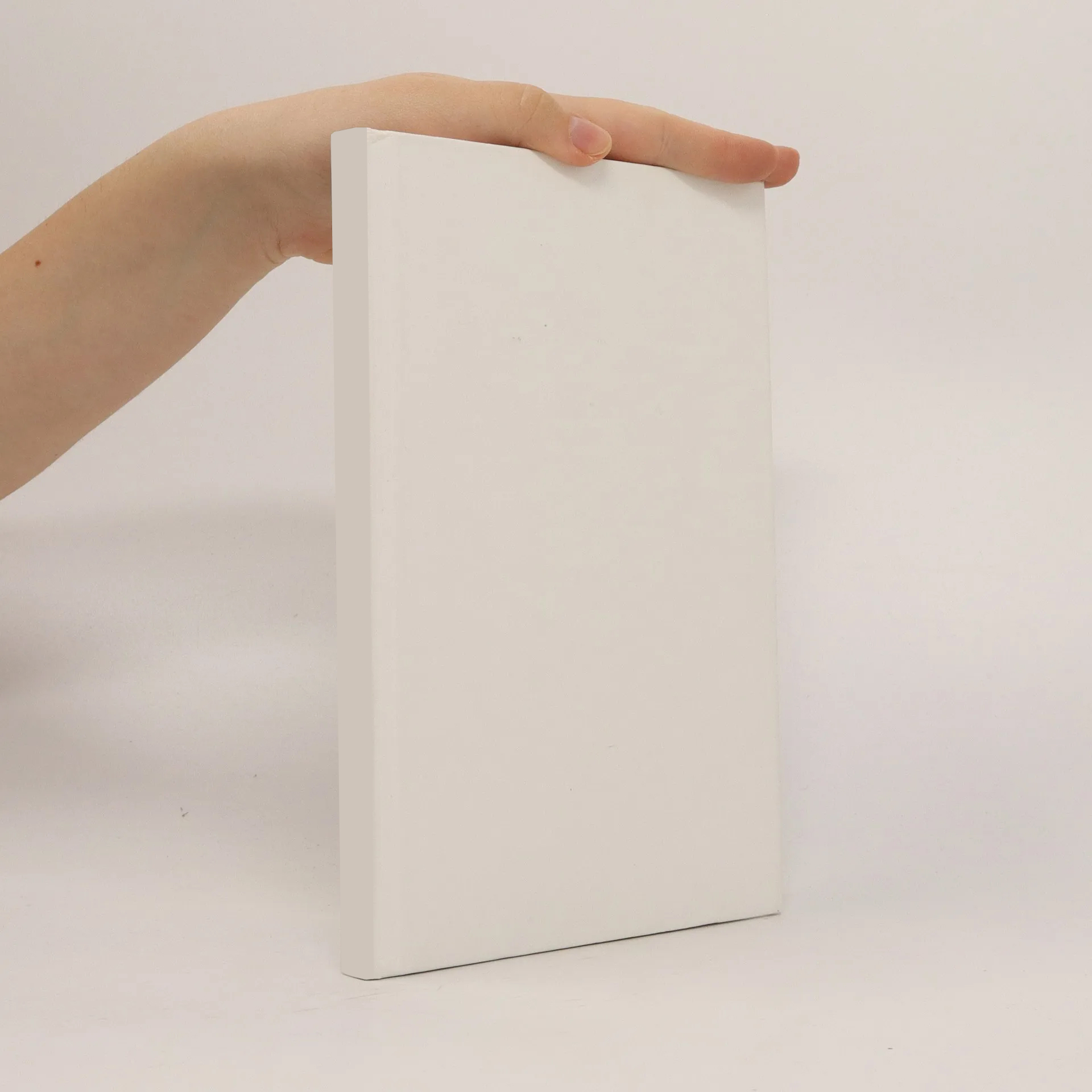
Parametry
Více o knize
The focus of the present work is set on correlation in the setting of financial markets. More precisely, the chief interest lies in efficient estimation of the correlation between returns of asset pairs, which is achieved by using additional information on the price processes. The meaning of additional information is twofold – on the one side it means information on daily highs and lows in addition to daily open and close prices, on the other side it refers to high-frequency data. Building on results in Rogers and Zhou (2008), we introduce a new construct - Balanced Excess Return (BER), which is the sum of the wicks (more precisely the balance of the wicks since the upper and the lower wick have a different sign) in the classical Japanese candlestick illustration of the open, high, low, close (OHLC) data. In the setting of a Brownian Motion (BM) model we show that the correlation of the BERs is intimately connected to the process correlation, which indicates their importance for the estimation of the latter. Using the BERs we derive efficient and robust correlation estimators. The improvement of the estimators suggested in this work over the ones given in Rogers and Zhou (2008) is largely due to the fact that the latter (implicitly) assume that the process variances are known. In order to shed some light on the pitfalls of this seemingly innocent assumption we set the stage by discussing correlation estimation in the normal model. After introducing the baseline BM model we analyse modern approaches in correlation estimation. We distinguish between two cases. In the first one only daily OHLC prices are available, whereas the second one deals with highfrequency data. We conduct extensive simulation studies to check the efficiency and robustness of the suggested estimators. In particular we study the effects of jumps and excess kurtosis as exhibited by Merton Jump diffusions, Symmetric Variance Gamma and Normal Inverse Gaussian processes as well as the effects of dependent increments as exhibited by Ornstein-Uhlenbeck processes. The practical importance of the suggested estimators is emphasized in an empirical study on their statistical properties and, subsequently, in an application in the portfolio selection field.
Nákup knihy
Estimating correlation using intraday price data in financial markets, Valentin Popov
- Jazyk
- Rok vydání
- 2013
Doručení
Platební metody
Navrhnout úpravu
- Titul
- Estimating correlation using intraday price data in financial markets
- Jazyk
- anglicky
- Autoři
- Valentin Popov
- Vydavatel
- Shaker
- Rok vydání
- 2013
- ISBN10
- 3844023224
- ISBN13
- 9783844023220
- Kategorie
- Skripta a vysokoškolské učebnice
- Anotace
- The focus of the present work is set on correlation in the setting of financial markets. More precisely, the chief interest lies in efficient estimation of the correlation between returns of asset pairs, which is achieved by using additional information on the price processes. The meaning of additional information is twofold – on the one side it means information on daily highs and lows in addition to daily open and close prices, on the other side it refers to high-frequency data. Building on results in Rogers and Zhou (2008), we introduce a new construct - Balanced Excess Return (BER), which is the sum of the wicks (more precisely the balance of the wicks since the upper and the lower wick have a different sign) in the classical Japanese candlestick illustration of the open, high, low, close (OHLC) data. In the setting of a Brownian Motion (BM) model we show that the correlation of the BERs is intimately connected to the process correlation, which indicates their importance for the estimation of the latter. Using the BERs we derive efficient and robust correlation estimators. The improvement of the estimators suggested in this work over the ones given in Rogers and Zhou (2008) is largely due to the fact that the latter (implicitly) assume that the process variances are known. In order to shed some light on the pitfalls of this seemingly innocent assumption we set the stage by discussing correlation estimation in the normal model. After introducing the baseline BM model we analyse modern approaches in correlation estimation. We distinguish between two cases. In the first one only daily OHLC prices are available, whereas the second one deals with highfrequency data. We conduct extensive simulation studies to check the efficiency and robustness of the suggested estimators. In particular we study the effects of jumps and excess kurtosis as exhibited by Merton Jump diffusions, Symmetric Variance Gamma and Normal Inverse Gaussian processes as well as the effects of dependent increments as exhibited by Ornstein-Uhlenbeck processes. The practical importance of the suggested estimators is emphasized in an empirical study on their statistical properties and, subsequently, in an application in the portfolio selection field.