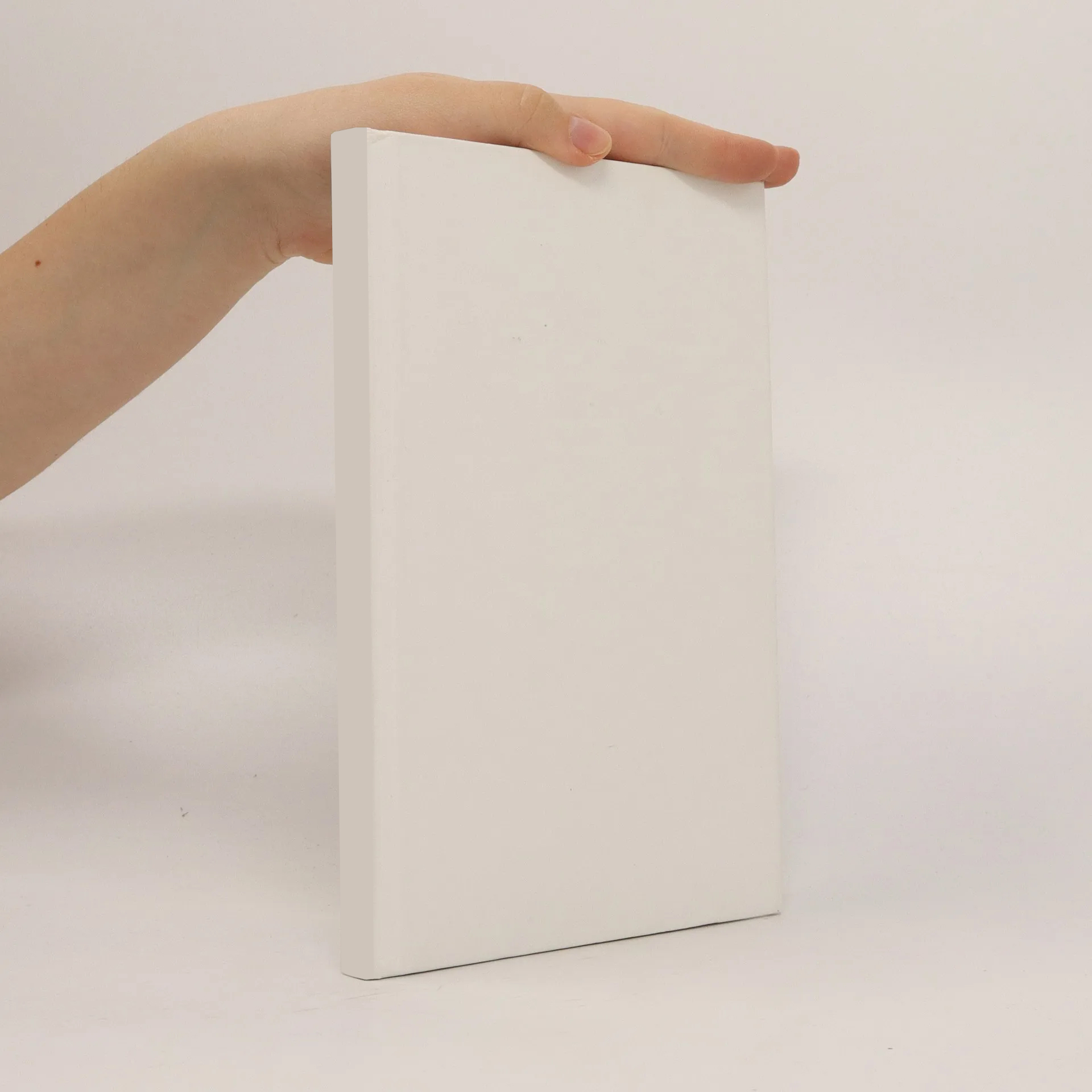
Schätzen der Klassenzugehörigkeitswahrscheinlichkeit zur Definition des Arbeitsbereichs von chemieinformatorischen Klassifikationsmodellen
Autoři
Parametry
Kategorie
Více o knize
This study analyses several regression- and classification techniques regarding their ability to estimate class-probabilities precisely. Furthermore the effect of the calibration (with use of logistic regression) and the influence factors: accuracy, correlation structure and data-set-size were analyzed. Class-probability-estimates can be used to define an applicability domain for a regression- or classification-model. The definition of an applicability domain by using class-probability-estimates (Reject Option) was compared to the approach of the Conformal Predictors. In summary, all studied techniques (Random Forests, Random Forest Regression, Support Vector Machines, Support Vector Regression, K-Nearest-Neighbor, Partial Least Squares Discriminant Analysis, Sparse Partial Least Squares Regression, Ridge Regression, Elastic Net, Least Absolute Shrinkage and Selection Operator), except Linear Discriminant Analysis, Neural Networks and Naïve Bayesian Classifier benefit from calibration with logistic regression. The accuracy and the correlation structure have the strongest impact. The stability of class-probability-estimates improves by generating hetero-ensembles. Good calibrated class-probability-estimates are able to define an applicability domain in a very efficient way, compared to the conformal predictor.
Nákup knihy
Schätzen der Klassenzugehörigkeitswahrscheinlichkeit zur Definition des Arbeitsbereichs von chemieinformatorischen Klassifikationsmodellen, Miriam Mathea
- Jazyk
- Rok vydání
- 2018
Doručení
Platební metody
2021 2022 2023
Navrhnout úpravu
- Titul
- Schätzen der Klassenzugehörigkeitswahrscheinlichkeit zur Definition des Arbeitsbereichs von chemieinformatorischen Klassifikationsmodellen
- Jazyk
- německy
- Autoři
- Miriam Mathea
- Vydavatel
- Cuvillier Verlag
- Rok vydání
- 2018
- ISBN10
- 3736997221
- ISBN13
- 9783736997226
- Kategorie
- Počítače, IT, programování
- Anotace
- This study analyses several regression- and classification techniques regarding their ability to estimate class-probabilities precisely. Furthermore the effect of the calibration (with use of logistic regression) and the influence factors: accuracy, correlation structure and data-set-size were analyzed. Class-probability-estimates can be used to define an applicability domain for a regression- or classification-model. The definition of an applicability domain by using class-probability-estimates (Reject Option) was compared to the approach of the Conformal Predictors. In summary, all studied techniques (Random Forests, Random Forest Regression, Support Vector Machines, Support Vector Regression, K-Nearest-Neighbor, Partial Least Squares Discriminant Analysis, Sparse Partial Least Squares Regression, Ridge Regression, Elastic Net, Least Absolute Shrinkage and Selection Operator), except Linear Discriminant Analysis, Neural Networks and Naïve Bayesian Classifier benefit from calibration with logistic regression. The accuracy and the correlation structure have the strongest impact. The stability of class-probability-estimates improves by generating hetero-ensembles. Good calibrated class-probability-estimates are able to define an applicability domain in a very efficient way, compared to the conformal predictor.