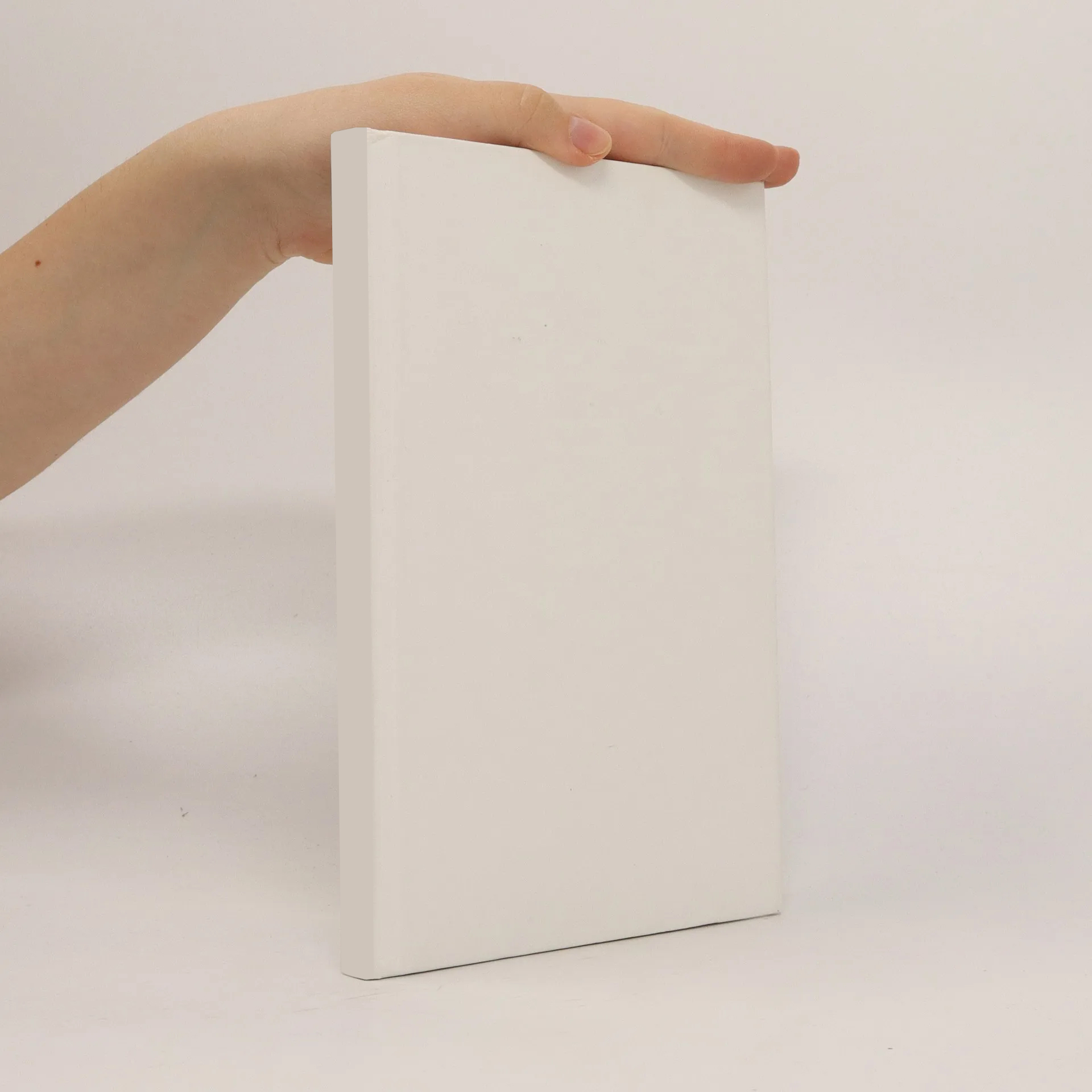
Robust multi-stage nonlinear model predictive control
Autoři
Více o knize
Model Predictive Control (MPC) has become one of the most popular control techniques in the process industry mainly because of its ability to deal with multiple-input-multiple-output plants and with constraints. However, its performance can deteriorate in the presence of model uncertainties. In the last years, the development of robust MPC techniques has been widely discussed, but these were rarely applied in practice due to their conservativeness or their computational complexity. This thesis presents multi-stage nonlinear model predictive control (multi-stage NMPC) as a promising non-conservative robust NMPC control scheme. The approach is based on the representation of the evolution of the uncertainty by a scenario tree. It leads to non-conservative robust control of the plant because it takes into account explicitly that new information (usually present as measurements) will become available at future time steps and that the future control inputs can be adapted accordingly, acting as recourse variables. Different aspects of the proposed multi-stage NMPC scheme are studied in detail in this thesis. Firstly, the approach is analyzed theoretically, including a formulation that guarantees stability and constraint satisfaction. Secondly, an efficient implementation is described, which is necessary to deal with one of the challenges of the presented method: The size of the resulting optimization problems. Thirdly, novel algorithms and modifications are proposed to enhance its performance and capabilities. The method is evaluated using examples from the chemical engineering field. Several simulations and real experiments show that multi-stage NMPC is a promising strategy for the optimizing control of uncertain nonlinear systems subject to hard constraints.