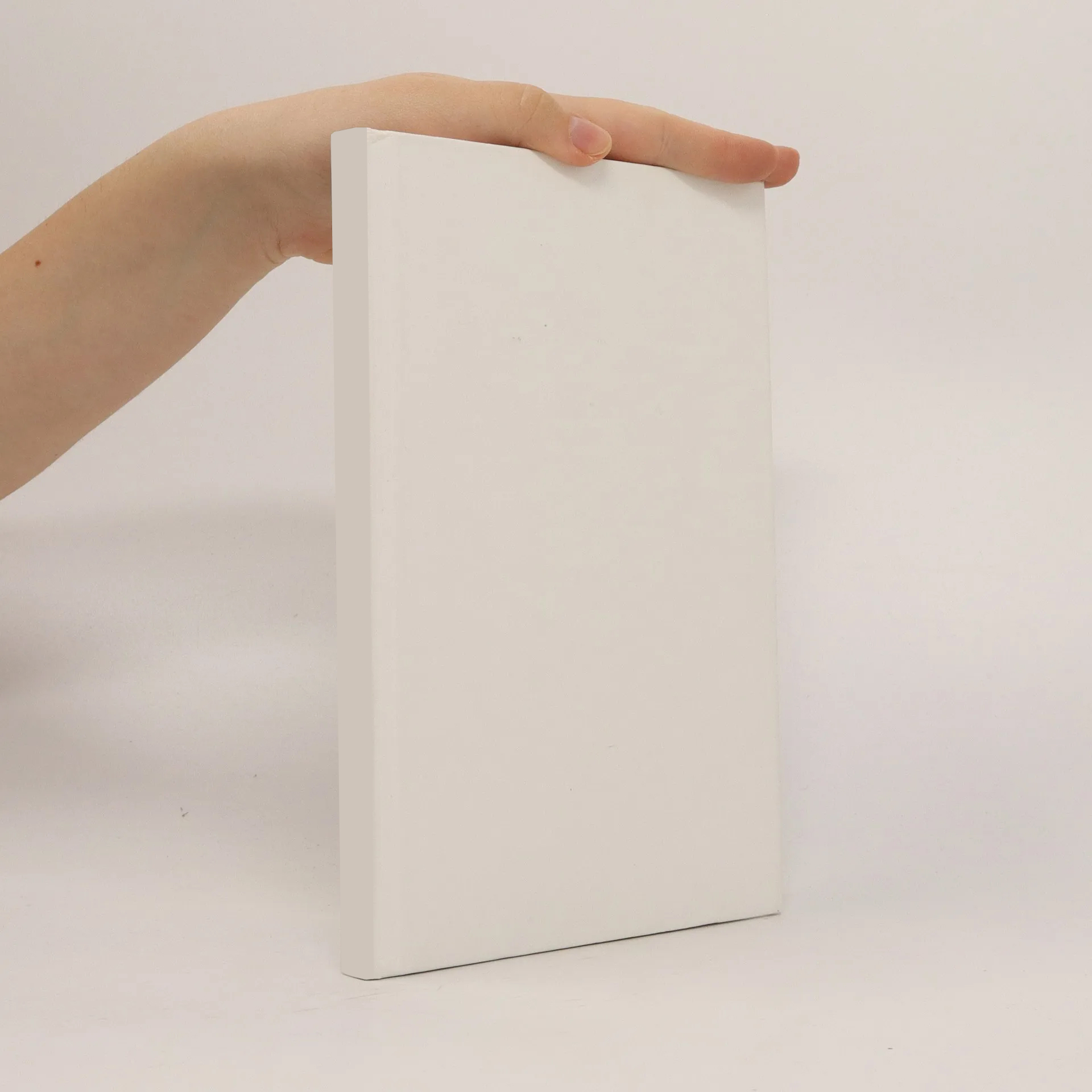
Speech enhancement using data-driven concepts
Autoři
Více o knize
Speech communication frequently suffers from transmitted background noises. Numerous speech enhancement algorithms have thus been proposed to obtain a speech signal with a reduced amount of background noise and better speech quality. In most cases they are analytically derived as spectral weighting rules for given error criteria along with statistical models of the speech and noise spectra. However, as these spectral distributions are indeed not easy to be measured and modeled, such algorithms achieve in practice only a suboptimal performance. In the development of state-of-the-art algorithms, speech and noise training data is commonly exploited for the statistical modeling ofthe respective spectral distributions. In this thesis, the training data is directly applied to train datadriven speech enhancement algorithms, avoiding any modeling of the spectral distributions. Two applications are proposed: (1) A set of spectral weighting rules is trained from noise recordings in the target environment and stored as a look-up table, indexed by the a posteriori and a priori signal-to-noiseratio (SNR) estimates. Among these weighting rules, the best performance is achieved through a new optimization criterion by averaging ideal gain instances yielding a frequency-individual ideal gain averaging (IGA) estimator. (2) The second application is about an a priori SNR estimator using neural networks, which are trained under white noise signals. Evaluated for automotive, street, and office environments, both data-driven approaches apparently show less speech distortion, especially in speech onset, while maintaining a high level of noise attenuation in speech absence, if compared to state-of-the-art analytically developed noise reduction techniques. A new measurement test methodology for arbitrary speech enhancement or hands-free systems is also proposed. The internal processing of such a system is not always accessible. By modeling such a system as a black box, a signal separationtechniqueisproposedtoestimatethespeechandnoisecomponentsof the enhanced speech signal. These signal components can subsequently be used allowing a more accurate subjective or instrumental performance assessment of the hands-free system in terms of speech preservation and noise attenuation, respectively. In all evaluated scenarios, even in double-talk conditions, the new black box test methodology can achieve a similar relative performance to the existing white box type of test, where the internal processing such as the spectral weights is accessible. This test methodology has become a part of two International Telecommunication Union (ITU-T) Recommendations for handsfree communication in motor vehicles.