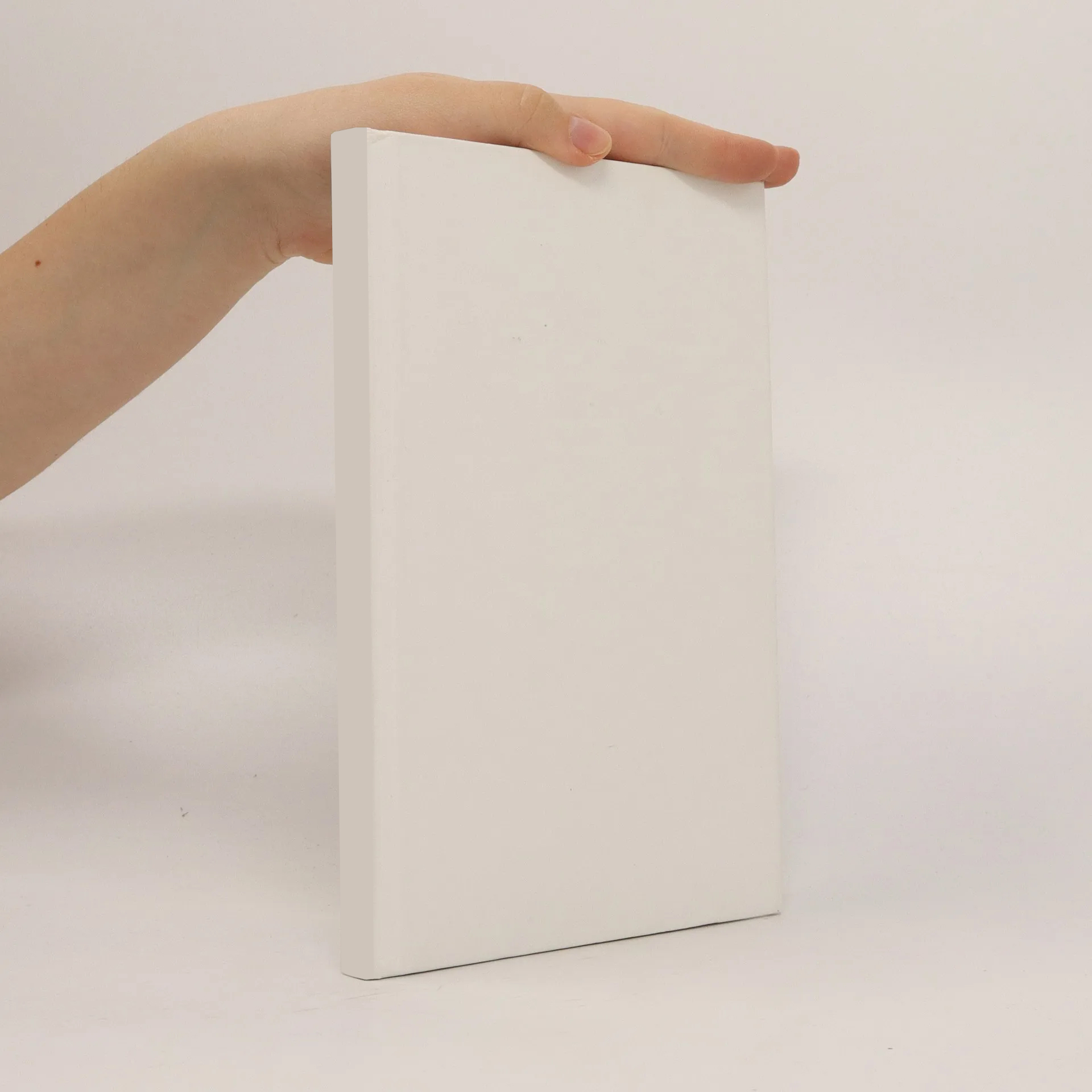
Learning based techniques for intercell interference coordination in LTE-advanced heterogeneous networks
Autoři
Více o knize
Drivenbynetworkdensificationandincreasingnumberofsmart-phones, tablets, andnetbooks, mobileoperatorsarecompelledtofindviablesolutionstoimprove their network performance in a cost-effective manner, and tap into new revenue streams. Heterogeneous network deployments combining a range of cell sizes (femto, pico, relays) with existing macrocells are expected to become cornerstones for future cellular networks and beyond, aiming at substantially higher data rates and maximum spatial reuse by decreasing the distance between transmitters and receivers. However, these future generation wireless networks face many challenges out of which intercell interference is crucial. It is the key requirement of small cells not to interfere the existing macrocellular system while at the same time guaranteeing their own receivers’ quality of service requirements. Hence, attention has been recently turned into self-organizing networks that permit improvements in system performance by automated intercell interference control and reductions in operational expenses. By considering these self-organizing capabilities, the main focus of this thesis is onlearningbasedintercellinterferencecoordinationtechniquesinheterogeneous networks overlaid by small cells (femtocells and picocells). While femtocells are modeled in a totally decentralized manner without any information exchange withthemacrocellularnetwork, picocellsexchangeinformationbyusingexisting interfaces. Both types of heterogeneous networks are modeled as multi-agent systems, in which intercell interference coordination is performed based on reinforcement learning. In case of femtocells, decentralized Q-learning algorithms are analyzed that are known to converge to optimal policies. However, their convergence is very slow for practical wireless systems. Improvements in terms of Fuzzy Q-learning are applied to the femtocell scenario. Further convergence improvements for Q- learning are provided by using estimations in the initialization phase of the algorithm. Finally, a decentralized multi-armed bandit reinforcement learning algorithm is introduced, that fits best with the small cells’ key requirement and converges within a few iterations, which makes the algorithm interesting for practical systems. In picocell based heterogeneous networks, Q-learning is applied as a two level game, inwhichboth, picocells andmacrocells, performlearninginordertomanage intercell interference and implicitly coordinate their transmissions. The proposed solution is adaptive and self-organizing in nature. Picocells autonomously optimizetheirstrategieswithloosecoordinationwiththemacrocellularnetwork. Additionally, a satisfaction equilibrium based intercell interference coordination technique is proposed enabling picocells to guarantee a minimum quality of service level at lower complexity. Finally, the proposed approaches are validated in a comprehensive Long Term Evolution standard conform system level simulator.